ML for the business today
Artificial intelligence (AI) usually means machine learning (ML) and other related technologies used for business.
Of course this is not what the original meaning was supposed to be, but we are talking about business reality here, so we simplify and use AI.
It has many business purposes which have become mainstream. Data and extracting valuable information from it has become critical for successful business operations and planning. That’s not what AI only has to offer, but let’s start with the most common examples, then we can move on to the main topic – generative AI.
Most of the examples can be classified into various types of pattern recognition and classification.
Client segmentation
Who are your clients? What are the target groups for your ads and marketing campaigns? How would they respond to the promotions and advertisements?
How do you predict that? Machine learning (ML) is a great help here when discovering the invisible rules and relationships in data.
→ Read more about Natural Language Processing: Tasks and Application Areas
Sentiment analysis
Based on text, voice analysis, image analysis, web activity and other data, the algorithms decide what the opinion is of the person towards the products and quality of services.
→ Explore Avenga experience in Sentiment analysis. Google Natural Language Processing vs Custom Algorithm
Fraud detection
With billions of transactions per day, it’s impossible for humans to detect illegal and suspicious activities. Fraud detection has been an automated process for many years already. The predefined algorithms and rules detected millions of illicit transactions.
But, the adversaries are getting smarter all the time. So Machine Learning (ML) techniques are being used extensively to detect problems for which there’s no formula defined.
Trend analysis
It’s hard to predict the future. There are well-known algorithms for trends analysis that the mathematicians have known for tens of years and they are still being used today.
But what about variables and dependencies of which existence and characteristics we have no clue?
Again, machine learning (ML) techniques help to discover more depth in the data than traditional mathematical analysis.
Download our free eBooklet
Security threats detection
The digital economy is under constant attack from hackers, who steal personal and financial data. Even perfect security systems with thousands of known threat detection rules are not future proof and the adversaries continue to work on new methods of attacks and will inevitably outsmart these security systems.
Machine learning (ML) is of great help here as well, as it can detect suspicious behavior without predefined rules and it can discover rules which were not known when the attack comes.
Better grammar and spelling
Better grammar and spelling is something we use everyday without even thinking about. Definition based rule engines are augmented or even replaced by machine learning (ML) algorithms and they have proved to be more effective and accurate than previous ones.
Passive AI
These are very useful examples, so I’ll call them passive AI – analyzing the existing data and generating output and helping to make decisions or even making them automatically.
Creative generative AI
But what about AI creating things like images, movies, text, or voice?
How come? Isn’t that limited for humans alone?
Let us go quickly through some well known examples of generative AI.
Image processing
All of us remember scenes from the movies when someone says “enhance, enhance” and magically zoom shows fragments of the image. Of course it’s science fiction, but with the latest technology we are getting closer to that goal.
Now the typical use case is the intelligent upscaling of low resolution images to high resolution images using complex AI image generation techniques.
It’s now part of modern image processing softwares, such as Photoshop.
But… your modern TV also uses this technology. ML based upscaling for 4K, as well as FPS, enhance from 30 to 60 or even 120 fps for smoother videos.
The same applies to computer games which can upscale the resolution to 4K while maintaining high frames per second based on lower resolution textures. The results are impressive, much better than from traditional techniques, and textures are sharp and look natural.
Low resolution image
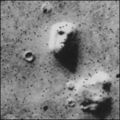
Image size 8x
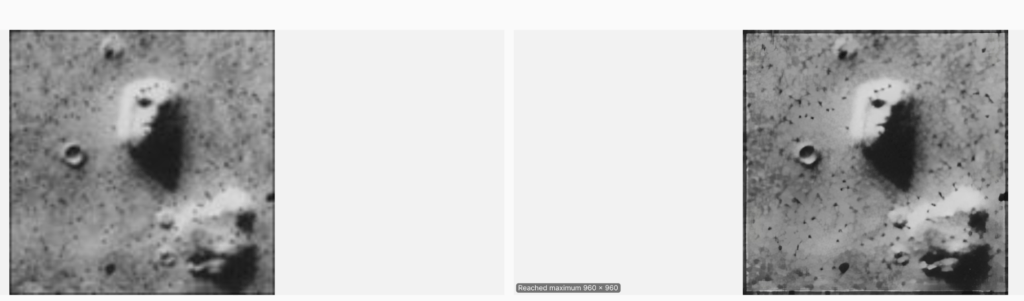
You can see the results achieved with traditional upscaling and after.
Movie restoration
We can see right now how ML is used to enhance old images and old movies by upscaling them to 4K and beyond, which generates 60 frames per second instead of 23 or less, and removes noise, adds colors and makes it sharp.
The results are impressive, especially when compared to the source images or videos, that are full of noise, are blurry and have low frames per second.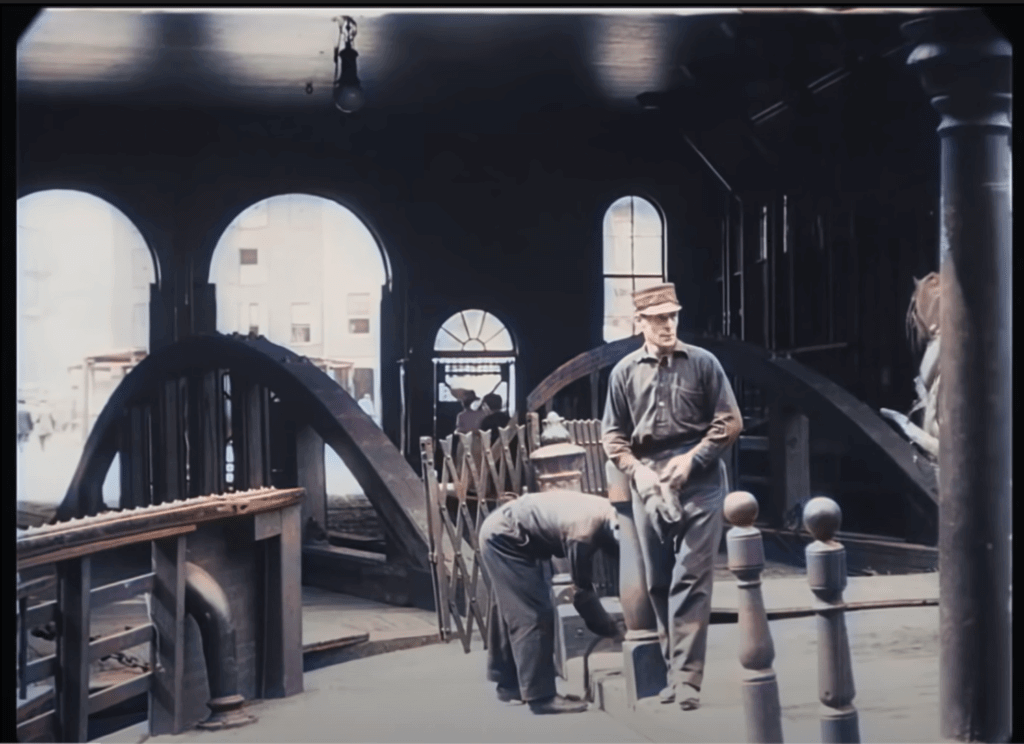
Screenshoot from “A Trip Through New York City in 1911“
In fact, the processing is a generation of the new video frames, which are based on the existing ones and tons of data to enhance human face details and object features. It’s not something that we have known for tens of years like traditional color enhancement or sharpening algorithms.
Super efficient video conferencing
NVIDIA announced a new ML based method for compressing video called Maxine used for teleconferences, that reduces the required bandwidth more than ten times, in other words, it enables ten times more people to attend the conference at the same time.
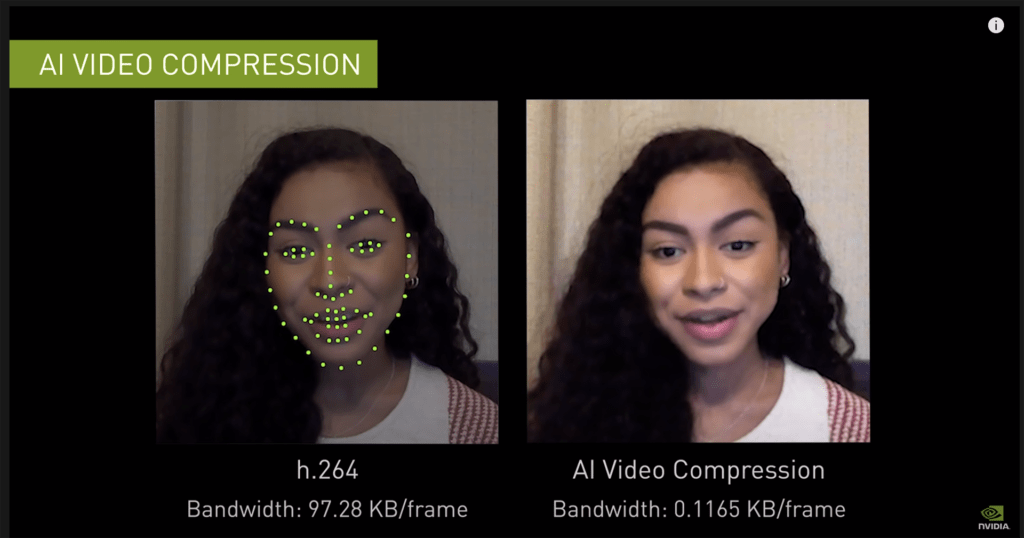
Screenshot from “AI-Powered Video Conferencing with NVIDIA Maxine”
This idea is completely different from the traditional MPEG compression algorithms, as when the face is analysed, only the key points of the face are sent over the wire and then regenerated on the receiving end.
Image generation for marketing
Photo sessions with real physical human models are expensive and require lots of logistical effort. There is also a complex law behind this activity, such as copyrights, etc.
But what about using ML to generate human images, and then using them in advertisements (i.e. web banners)?
Yes, it has already been done commercially.
If you want to see it for yourself, there are web pages with images of people who never existed.
https://www.thispersondoesnotexist.com/
Another website has more than two million photos, royalty free, of people who never existed but look like real people. You can select different parameters to get images that fit the specific criteria, and all this is generated by AI; none of these people even exist.
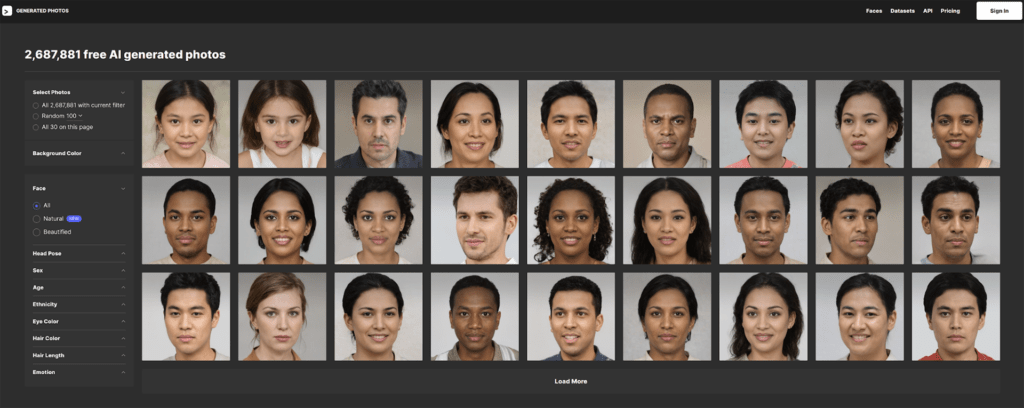
https://generated.photos/
(Sample image of the people who do not exist)
Writing articles and stories
With the advancements of technology, such as the famous GPT-3 which we covered in a different article, many people are simply stunned.
The text they read looks like it’s coming from a professional writer, as it’s interesting and the style is very human. The problem is that the contents may not be true and could be completely misleading. But still, it’s very impressive.
There are already attempts to use text generation engine’s output as a starting point for copywriters. In our case we did an interview with AI and it sounded really interesting and natural.
→ Avenga experience in Chatting about the future of AI with…the GPT-3 driven AI
Google Docs has a feature that attempts to automatically augment text with AI generated content.
Writing code
We have discussed before how AI is helping developers now.
→ Explore AI knock-knock-knockin’ on developers’ door
All modern IDEs contain advanced code generation tools and refactoring tools, and the machine learning (ML) techniques are also used here. It’s still a long way off to replacing developers, but now AI is a great help in improving the efficiency of coding and refactoring.
Biochemistry and drug discovery
Neural networks can generate multiple proteins very fast and then simulate the interactions with various molecules to discover drugs for different diseases. In 2019, substances generated by AI were tested on mice.
Our partners from the pharma industry hope the future is in AI generated drug candidates and simulations that use the petabytes of data they already have and continue to generate. It’s all for a very important reason: to discover effective and safe drugs faster and more efficiently.
Fake videos and images
There is news, almost every month, about a new scandal related to fake images, fake news, or fake videos whose intention is to fool people into believing fake stories and making wrong decisions, including voting decisions. Or, at least to humiliate famous people with fake nudes, putting false words in their mouths, etc.
With the improvements of AI generative technologies it’s become a serious problem. Static 2D images are the easiest to fake, but today we face the new threat of fake videos.
For instance neural networks can generate a video of a person speaking based on a single photograph!
What are the countermeasures?
There are AI techniques whose goal is to detect fake images and videos that are generated by AI. So we can talk about an AI vs. AI war. The accuracy of fake detection is very high with more than 90% for the best algorithms. But still, even the missed 10% means millions of fake contents being generated and published that affect real people.
→Read about Human digital twins. What are they and Why are they?
So, there’s strong criticism of using AI to fix problems created by other AI in the first place.
Generative AI techniques
Let’s quickly review the most popular techniques for generative AI.
Generative adversarial networks (GANs)
The idea is that two networks are used. One is generating (for instance images) while the second is verifying the results, for instance if the images are natural and look true.
In other words, one network generates candidates and the second works as a discriminator. The role of a generator is to fool the discriminator into accepting that the output is genuine.
By learning, both become better at what they do. The generator generates better results and the discriminator gets better at detecting ‘unnatural’ results.
The discriminator is usually a convolutional network (CNN) and the generator is the opposite, a deconvolutional neural network.
This is what learning looks like:
GANs are not the only approach, but also Variational Autoencoders (VAEs) and PixelRNN (example of autoregressive model).
Known limitations and issues
We all admire how good the creations coming from ML algorithms are but what we see is usually the best case scenario. Bad examples and disappointing results are nothing interesting to share about in the most popular publications. Admitting that we are still at the beginning of the generative AI road is not as popular as it should be. The progress is definitely visible, but the hype is always louder and stronger.
→ Explore Is Deep Learning hitting the wall?
GANs are unstable and hard to control, and they sometimes do not generate the expected outputs and it’s hard to figure out why. Controlling the behavior of these types of models is very difficult. When they work, they generate the best images; the sharpest and of the highest quality compared to other methods.
The ML scientists work on solutions for the known problems and limitations, and test different solutions, all the while improving the algorithms and data generation.
Future of generative AI
There will be more generative AI applications in the future, that’s for sure.
The cost of generating images, 3D environments and even proteins for simulations is much cheaper and faster than in the physical world.
This is the start of another disruption and even today companies are selling these photos. Modelling companies have started to feel the pressure and danger of becoming irrelevant.
As trust is becoming the most important value of today, fake videos, images and news will make it even more difficult to learn the truth about our world.
There will be stronger regulations, penalties and improved fake detection algorithms.
Unfortunately, despite these and future efforts, fake videos and images seem to be an unavoidable price to pay for the benefits we are expected to get from generative AI in the near future.
There’s a lot your business can benefit from with the current AI technology. Our data science team is excited about bringing the latest in machine learning to our customers to help them with real life business problems.