What kind of technology can be considered as the most promising and useful for business? Artificial Intelligence, Internet of Things, and big data? It depends on the industry specifics of a particular company and the processes that need to be improved, you say. And you are right. But does it really matter which innovations are introduced into the business to transform it, if the IT solutions’ related data is not prepared in any way and it looks like a kind of chaos? In any case, the company won’t get the results that it was expecting: customer dissatisfaction, inefficient resources management, and the inability to determine threats. Garbage at the entrance – garbage at the exit, as George Fuechsel, who used GIGO as a training method, said.
Strategic data management (quality assessment) is called Data Governance (DG), and practical (quality improvement) is called Data Management (DM). The fact that these concepts are not interchangeable was proclaimed by Michele Goetz, Forrester Chief Analyst. To make it easier for the audience to feel the difference between them, data officers from one of the biggest IT providers compared data processing and cooking. So, Data Management is like kitchen shelves that store products and all the cooking equipment, and Data Governance is the recipes/instructions that help to correctly dispose of a large number of ingredients and use the equipment to make a delicious dish out of them.
In other words, competent Data Management is impossible without a well-thought-out data management strategy. DM tools (i.e., ETL systems or MDM solutions) are standardized and they can be configured to work with specific data and processes. Data Government, on the contrary, is a unique plan that takes into account features and specifics of the particular company.
Each company should have its own Data Governance. According to Vimal Vel, Vice President of Dun & Bradstreet (a provider of data solutions), many of their customers are inspired by the idea of Data Governance and immediately begin to develop a strategy, without paying attention to the preparation, which should be, definitely, the preliminary (zero) stage of Data Governance. As our own Avenga experience with data solutions and services implementation proves, no one can proceed without it. At the beginning, you need to understand the stage of the business regarding DG and what you’d like to get after a certain time – most of the data that is being worked on as part of the strategy should be related to business performance.
Surveys show that many companies aren’t clear in considering strategic data management. In particular, only 48% of survey participants, conducted by First San Francisco Partners, had a program for their information processing. Meanwhile, there are several understandable principles that the Data Governance strategy is based on:
1. Provide data availability.
Neither “smart” system will work with 100% efficiency if it doesn’t have access to the necessary information. But here we must not forget about confidentiality. When drawing up a DG strategy, it is vital to understand which information you can provide to specialists for investigation and what data has to be anonymized.
2. Users should operate with consistent data.
The case when business employees work with one version of the data and the sales department with other data, can cause that as a result, conclusions about similar processes will be completely different. In this case, it would be difficult for business management to figure out who is right and whose recommendations should be accepted.Therefore, it is important that employees work with consistent data from a single source. Now companies have two options. The first is to set up a transparent data synchronization process between different repositories. The second is to place all the information in a single repository, for example, a Data Lake. This allows you to store both structured and raw data in different formats, not only with documents but also with media files such as video, audio, etc.
3. Understand what to collect and what to throw away.
Do not collect useless ‘garbage’. The use of irrelevant or outdated data for making decisions is unlikely to increase the company’s competitiveness.A data management strategy suggests that only actual and relevant data should be placed in the repository. As we remember, the plan is always individual; therefore, for each company the compliance criteria will be different. Do not turn your data lake into a swamp and set up processes to get rid of unnecessary information in time.→Explore Avenga Strategy Consulting ServicesOf course, permanently deleting data that does not meet the criteria is optional. You can put them in the archive, but first, it’s important to categorize everything carefully. If archive information is ever needed, finding it without a detailed description will be difficult.
4. Keep your data safe.
Any system can ever fail and all the information accumulated in it will disappear. Trust IT, but don’t forget about backups – another important principle.
5. Do not forget about security.
Meantime, the rules for handling data, including personal data, have become stricter and non-compliance can lead to fines and destroy the company’s reputation. Data Governance assumes that the business has clear instructions on how to protect information from theft or unauthorized use. Even if the company is small, you should not think that it is not interesting for cybercriminals – on the contrary, hacking that system will be easier than getting into the storage of a large corporation.→ Read about data validation testing techniques and approaches to help you ensure the data you deal with is correct and complete.
Main principles of data governance
Many organizations want to share data sets across the enterprise, but taking the first steps can be challenging. These challenges range from purely technical issues, such as data formats and APIs, to organizational cultures in which managers resist sharing data they feel they own. Data Governance is a set of practices that enables data to create value within an enterprise. When launching a data governance initiative, many organizations choose to apply best practices, such as those collected in the Data Management Association’s Body of Knowledge (DAMA-BOK). While these practices define a desirable end state, attempting to apply them broadly across the company as a first step can be disruptive, expensive, and slow to deliver value. Here are six main DG principal things, described by John Klein in the “Six Things You Need to Know About Data Governance” publication.
1. A data set produces benefits only when it is used to make decisions.
If we apply best practices, for example, clean a data set, publish its schema, assign a data steward, and layer on an open API, but nobody ever uses the data set, then we have not produced any direct benefits. Decisions and actions produce benefits and until we use a data set to support decision-making, it is just incurring costs. (We acknowledge that a data set that is ‘ready to go’ has option value, but that should not be your initial data governance focus).
2. Value ≣ ∑benefits – ∑costs The value of a data set is the sum of the benefits it produces (that is, the benefits of the decisions that the data set supports) minus the sum of the costs to use the data set. Obviously, we want this value to be positive.
3. Data has a value chain.
The value chain for a data set has four moving parts, as shown here:
The first part is the data Producer, which could be a sensor, open-source feed, or another system. Next, a Publisher acquires the data set, stores it, and makes it accessible within the enterprise. A Consumer develops a decision support application or analytic that uses the data set, and a Decision-Maker uses the application to make decisions. There are variations, where a single entity plays more than one role. For example, the Producer may also publish, or the Consumer may also be the Decision-Maker.For our scope of data sharing within an enterprise, in almost all cases, the first three parts only incur cost and benefits are only produced by the Decision-Maker.We are going to focus on the Publisher and Consumer. In many cases, the Producer is outside the scope of our authority and the Decision-Maker is executing a business or mission process that is also outside of our authority. We’ll focus on things we can control.In the case where there is just a single Publisher and a single Consumer, things are easy to manage. There is a single value chain. The data set may need to be reformatted, cleaned, or enriched, but usually, the Publisher and Consumer can agree about how to split the costs associated with using the data set.On the other hand, when there are multiple Consumers, each with different needs from the data set, the problem becomes more challenging. We have the potential for duplication of effort by Consumers, for example, if each Consumer must remove duplicate records in the data set. Alternatively, the Publisher may negotiate separate agreements with each Consumer to deliver a customized version of the data or a different API, which duplicates the work of the Publisher. These costs incurred from duplication of effort will reduce the value we produce from using the data. To minimize these costs, we need to take a broader perspective.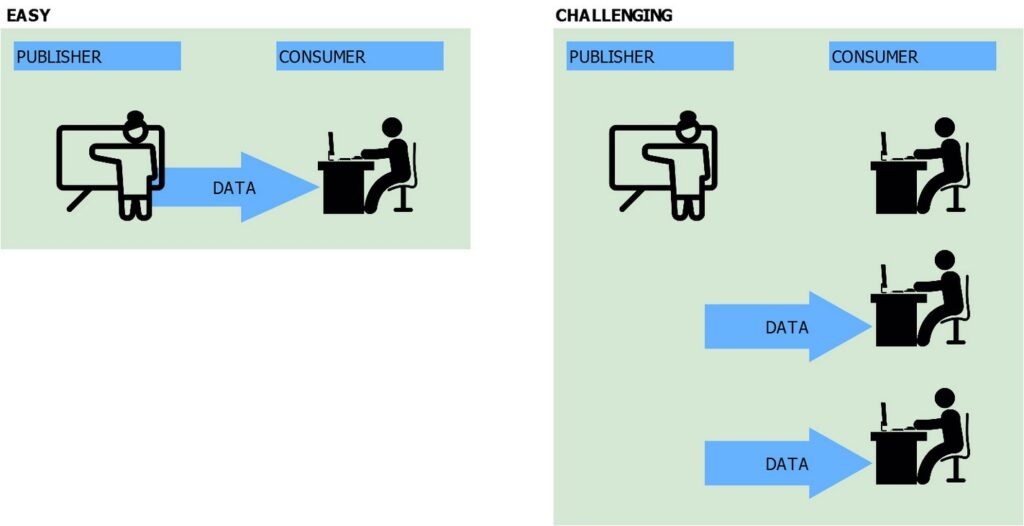
4. Governance constrains the data publisher to help the data Consumers.
Governance assigns responsibilities and limits freedom. In this case, we constrain the Publisher to deliver the data set in a way that is best for all Consumers. We do this by analyzing the value chain through all of the Consumers and allocating responsibilities (and hence, costs) between the Publisher and Consumers to maximize the total value produced by all uses of the data set.Governance manifests in an organization’s Enterprise Architecture as standards, patterns, and policies and is reviewed as part of the organization’s software engineering process–for example, at phase gate reviews.Governance needs authority as making rules that nobody follows incurs only costs, with no offsetting benefits, and hence produces negative value.Constraining the Publisher may reduce the Publisher’s costs, for example, by reducing the types of interfaces, restricting backward compatibility requirements for an interface, or restricting technology options. However, the constraints usually increase the Publisher’s costs, for example, by requiring a schema transformation, increased data quality, or higher availability. These improvements help Consumers reduce their cost to use the data. The improvements also reduce duplication of work across all Consumers, thereby increasing total value.
5. Apply governance only when it increases value (benefits > costs).
We don’t need to govern every data set in the enterprise. In fact, if the enterprise has mostly one-to-one exchanges between a single Publisher and single Consumer, then investing in data governance may not be worthwhile because the costs will outweigh any benefits.
6. Focus your governance on the things that data Consumers want.
Governance constrains the Publisher. We should tailor those constraints for each data set. One data set may warrant significant investment in improving data quality, while another data set may simply need to be stored on a Hadoop cluster.To focus on what data Consumers want, a five-part Data Consumer Concerns framework has been created to categorize their concerns. The framework categories provide a checklist, and some typical questions were provided that a data Consumer would need to answer to effectively use a data set.
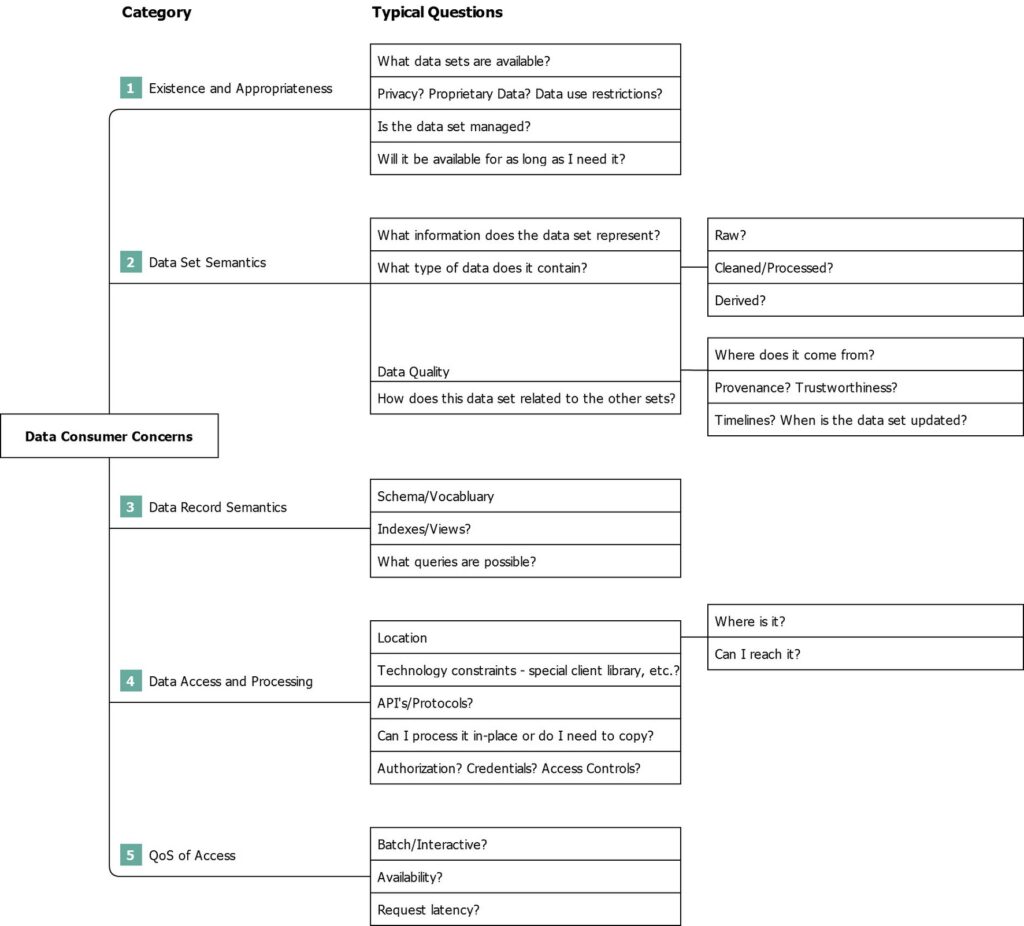
First, Consumers need to know what data is available and whether that data set is appropriate for their use:
- Are there restrictions on data use?
- Will the data set be available for as long as they need it?
The data set needs to pass these tests before moving to the next category.
The second category (Data Set Semantics) addresses concerns about the meaning of the complete data set:
- What information does it represent?
- Where did it come from?
- Does it depend on or complement other data sets.
The third category focuses on the meaning and structure of each record in the data set.
The fourth category covers concerns about accessing the data set, such as is it reachable, what are the interface protocols and APIs, and how is access controlled.
Finally, Consumers are concerned about quality of service. The data sets that they use must be delivered with availability and performance that is consistent with the requirements for the applications that they are building.
An enterprise data catalog is a mechanism to capture and communicate this information about data sets within the enterprise. The data catalog is a repository that contains information about the data sets (i.e., metadata) that are available in the enterprise. There are commercial products that implement metadata catalogs; however the initial version of the catalog can be implemented using any lightweight technology that supports searching or sorting, such as a wiki, SharePoint site, or even a shared spreadsheet. If you start with a lightweight implementation, you can decide what features and scale you need and migrate to a commercial product if needed.
→ Read how the business domain knowledge plays a vital role in providing software quality.
A playbook for data governance
Combining the six principles discussed above, the playbook for lightweight data governance can be described as follows:
Step 1: Identify your high-benefit decisions. These decisions might be infrequent but high impact, high frequency but low impact, or something in between.
Step 2: Identify the data sets that support your highest-benefit decisions.
Step 3: For each of those data sets identify the producer-consumer relationship. If it is a one-to-one relationship, then little or no governance may be needed. If it is a one-to-many or a many-to-many, then governance may increase value.
Step 4: What constraints should you impose on the Producer? How will data Consumers need to adapt? Use the Data Consumer Concerns framework described above to identify possible governance actions. At each point, balance costs and benefit to keep value positive.
Step 5: Repeat Steps 2, 3, and 4 for each high-benefit decision identified in Step 1.
Step 6: Periodically review your list of high-benefit decisions for changes and introduce or remove governance constraints using a data set value to guide decision making.
The data governance framework
A data governance framework is a set of data rules, organizational role delegations and processes aimed at bringing everyone in the organization onto the same page.
There are many data governance frameworks out there. As an example, we will use the one from The Data Governance Institute. This framework has 10 components; let’s discuss it in detail.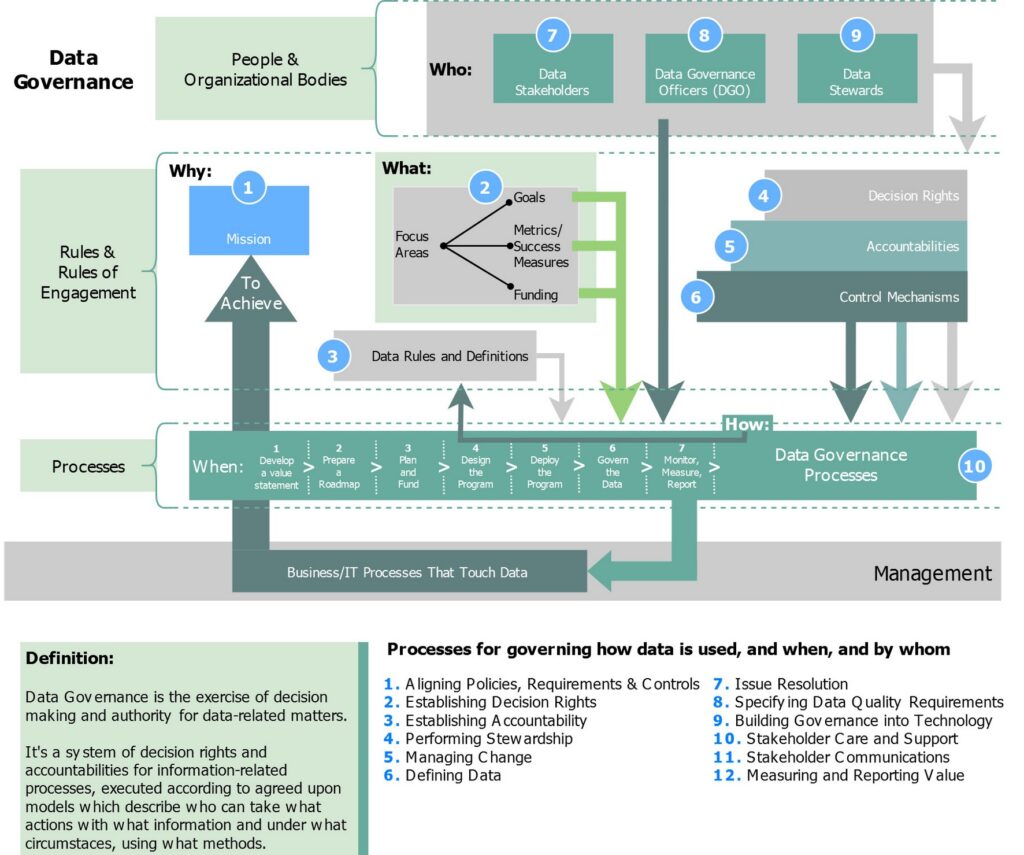
WHY:
The master data can be described by the way that it interacts with other data.
A mission and vision that states why Data Governance is essential within our organization. At best, this should be related to the business objectives of the enterprise. This should be endorsed by top management.
WHAT:
The short-term and long-term goals for the Data Governance program as well as the success criteria and their measurement. Often this should address the main pain points that exist in various lines of the business. This must be aligned with the funding and other involved line management.
Data rules and definitions in the form of data policies, data standards, and data definitions preferable as a business glossary, as well as how business rules transform into data rules. This should cover the data assets describing the core business entities essential to meeting the business objectives. The data governance office/team will work with data owners and data stewards to set this up.
- The decision rights that exist for managing the data assets in the day-to-day business. This will include what data stewards can decide and what must be escalated to a data governance committee or similar authority.
- The accountabilities and related responsibilities delegated within the organization. This can include a full RACI matrix with counsel and informed roles as well.
- The control mechanisms that are put into action in order to measure the adherence of data rules and achievements toward the defined goals. The mechanisms can be established within business processes, in IT applications, and as part of reporting.
WHO:
Engagement of data stakeholders in the roles of data owners, data stewards, data custodians, and others who are accountable and/or responsible must be consulted or should be informed.
The Governance Office / Team should be organized to support the cross-functional data governance structures and activities. It collects metrics and success measures and reports on them to data stakeholders. It provides ongoing stakeholder care in the form of communication, access to information, record-keeping, and education/support.
- Data stewards will play an essential part in enforcing data rules and resolve most issues before they become a major challenge. A typical responsibility for data stewards will be setting up the data quality measurements and following up on the trends in the data quality KPIs, and performing root cause analysis where thresholds are not met.
WHEN:
Last, but not least, a set of standardized, documented, and repeatable processes must be deployed with the right balance of enabling technology. The orchestration of data governance processes will ultimately determine the success – or failure – of your data governance framework and the ability to grow in data governance maturity.
The maturity model
Measuring your organization up against a data governance maturity model can be a very useful element in making the roadmap and communicating the as-is and to-be part of the data governance initiative and the context for deploying a data governance framework.
One example of such a maturity model is the Enterprise Information Management maturity model from Gartner, an analyst firm.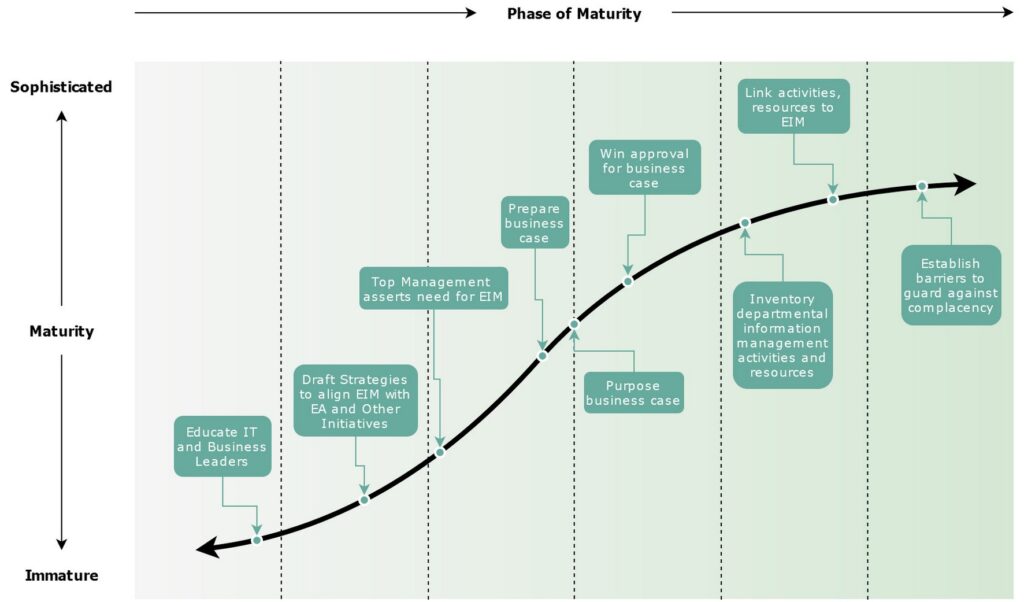
Most organizations will, at the beginning of a data governance program, find themselves in the lower phases of such a model.
Phase 0 – Unaware: This might be in the unaware phase, which often will mean that you may be more or less alone in your organization with your ideas about how data governance can enable better business outcomes. In this phase, you might have a vision for what is required but you need to focus on much humbler things such as convincing the right people in the business and IT about smaller goals around awareness and small wins.
Phase 1 – Aware: In the aware phase, where lack of ownership and sponsorship is recognized and the need for policies and standards is acknowledged, there is room for launching a tailored data governance framework addressing obvious pain points within your organization.
Phases 2 and 3 – Reactive & Proactive: Going into the reactive and proactive phases means that a more comprehensive data governance framework can be established covering all aspects of data governance and the full organizational structure that encompasses data ownership and data stewardship, as well as a Data Governance Office / Team, in alignment with the achieved and to be achieved business outcomes.
Phases 4 and 5 – Managed & Effective: By reaching the managed and effective phases your data governance framework will be an integrated part of doing business.
If your current data governance policies and procedures are your guidebook, the maturity model is your history book. It’s compiled from historical data based on a maturity assessment, which compares a company’s performance to established goals and benchmarks over a given period – a quarter, for example, or a year, or even five years. The model shows where you’ve been, which helps shape where you’re going.
While a “one-size-fits-all” approach doesn’t really work for a maturity model, an “if-the-shoe-fits” approach works well for many companies. Search for existing models, find one that’s close, and adjust it to meet your company’s needs. If the shoe doesn’t fit, it’s easy to change the size of the shoe. It’s not so easy to change the size of your foot.
Connection to MDM
Data Governance is the strategic approach. Master Data Management (MDM) is the tactical execution. That’s it. We’re good. You can go home now.
Not convinced? Ok. Don’t take our word for it. As promised, we’re back with Scott Taylor of MetaMeta Consulting. He has forgotten more about master data than most of us will ever know, so we’re happy to give him the last word.
“All enterprise systems need master data management,” Scott said at our Profisee 2019 kickoff event. “Marketing, sales, finance, operations. There is benefit everywhere, in enterprises of any size, in every industry, across the globe, at any point in their data journey.”
Master data is the most important data, Scott said, because it is the data in charge. It’s about the “business nouns”–the essential elements of your business: customers, partners, products, and services. Whatever your business is, that’s where master data lives and breathes. You may have the best governance plan on the planet but well-governed bad data is still bad data. It’s not going to help your business.
“Everybody is in the data business, whether they realize it or not,” Scott said. “Everything we touch turns to data. Business is transforming from analog to digital. No matter what your product is, data is your product. Business is changing because of data, and data is power.”
“With the right tools, you can harness that power right now.”
We couldn’t have said it better ourselves.
Data protection and data privacy
The increasing awareness around data protection and data privacy, for example, manifested by the European Union General Data Protection Regulation (GDPR), have a strong impact on data governance.
The terms of data protection by default and data privacy by default must be baked into our data policies and data standards especially when dealing with data domains as employee data, customer data, vendor data, and other party master data.
→ Explore more Essentially, Data is good. It’s the use cases that can be problematic
As a data controller, you must have full oversight over where your data is stored, who is updating the data, and who is accessing the data and for what purposes. You must know when you handle personally identifiable information and do it for legitimate purposes in the given geography, both in production environments and in test and development environments.
Having well-enforced rules for the deletion of data is a must too in the compliance era.
Best practices
On the one hand, you can learn a lot from others who have been on a data governance journey. However, on the other hand every organization is different and you need to adapt data governance practices all the way, starting from the unaware maturity phase to the nirvana in the effective maturity phase.
Nevertheless, please find below a collection of the 15 short best practices that will apply in general:
- Start small. As in all aspects of business, do not try to boil the ocean. Strive for quick wins and build up ambitions over time.
- Set clear, measurable, and specific goals. You cannot control what you cannot measure. Celebrate when goals are met and use this to go for the next win.
- Define ownership. Without business ownership, a data governance framework cannot succeed.
- Identify related roles and responsibilities. Data governance is teamwork with deliverables from all parts of the business.
- Educate stakeholders. Wherever possible use business terms and translate the academic parts of the data governance discipline into meaningful content in the business context.
- Focus on the operating model. A data governance framework must integrate into the way of doing business in your enterprise.
- Map infrastructure, architecture, and tools. Your data governance framework must be a sensible part of your enterprise architecture, the IT landscape, and the needed tools.
- Develop standardized data definitions. It is essential to strike a balance between what needs to be centralized and where agility and localization work best.
- Identify data domains. Start with the data domain that has the best ratio between impact and effort for growing the data governance maturity.
- Identify critical data elements. Focus on the most critical data elements.
- Define control measurements. Deploy these in business processes, IT applications, and/or reporting where it makes the most sense.
- Build a business case. Identify the advantages of growing data governance maturity related to growth, cost savings, risk, and compliance.
- Leverage metrics. Focus on a limited set of data quality KPIs that can be related to general performance KPIs within the enterprise.
- Communicate frequently. Data governance practitioners agree that communication is the most crucial part of the discipline.
- It’s a practice, not a project.
At Avenga, we craft digital experiences that define the success of your business. Being around for over 20 years, we have been exploring, experimenting and implementing data solutions and services to make data work for your business needs.
Links and sources
- DAMA Body of Knowledge: https://dama.org/content/body-knowledge
- Six Things You Need to Know About Data Governance by John Klein: https://insights.sei.cmu.edu/sei_blog/2017/06/six-things-you-need-to-know-about-data-governance.html
- Data Governance – What, Why, How, Who & 15 Best Practices by Profisee Tech Blog: https://profisee.com/data-governance-what-why-how-who/#a4
- Роман Баранов. Data Governance: зачем вам стратегия управления данными?: https://www.it-world.ru/tech/business/148814.html